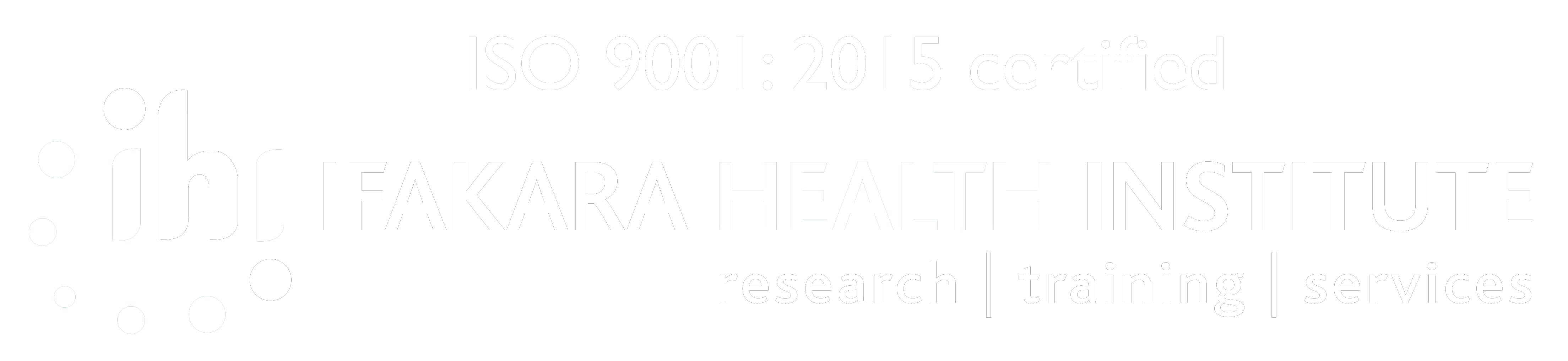
INNOVATION: Using AI technology to detect malaria
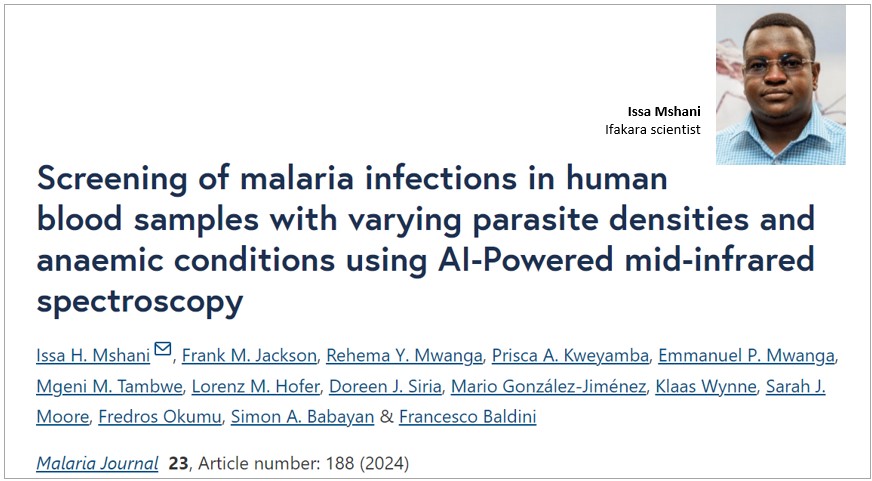
What if we could detect malaria more accurately and cheaply than ever before? Scientists from the Ifakara Health Institute have recently confirmed that this is possible using an AI-powered technique. The method can detect malaria infections with high (over 90%) accuracy, which is vital for the successful elimination of the disease.
In their paper published on the Malaria Journal, the scientists detail the application of the innovative method, which uses mid-infrared spectroscopy to detect malaria infections, offering a more sensitive and cost-effective alternative to existing tests.
“These findings suggest that the AI-driven mid-infrared spectroscopy approach holds promise as a simplified, sensitive and cost-effective method for malaria screening...” they wrote.
Describing the approach as “simple and user-friendly” the scientists demonstrated how the AI-powered, reagent-free, uses mid-infrared spectra from dried blood spots to accurately detect malaria infections across varying parasite densities and anaemic conditions.
The problem with current tests
Detecting malaria infection, especially at low densities, is vital for successfully eliminating the disease. However, there is a dilemma with existing methods; they are either inexpensive but poorly sensitive or sensitive but costly.
For example, while Rapid diagnostic tests (RDTs) have transformed malaria case management across Africa due to their practicality and affordability, they often lack sensitivity. Equally, more accurate methods like microscopy are costly and require skilled personnel.
The AI-powered technique addresses this issue by offering over 90% accuracy in detecting malaria infections as low as one parasite per microlitre of blood, a sensitivity unattainable by conventional RDTs and microscopy.
How the study was done
Conducted at Ifakara Health Institute’s laboratories in Bagamoyo, the study involved the use of mid-infrared spectroscopy and machine learning to analyze dried blood spots.
The scientists created various samples with different levels of malaria and anemia with blood obtained from 70 malaria-free individuals to mimic real-life conditions. AI was then trained to detect malaria in these samples.
The results were remarkable with the AI-powered technique able to detect malaria with over 90% accuracy, even at very low levels. The technique seamlessly transitioned to field applicability, achieving over 80% accuracy in predicting natural malaria infections in blood samples collected during the field tests in malaria-endemic villages in southeastern Tanzania.
Optimism for real-world applications
In conclusion, the scientists expressed optimism about the potential impact of their findings regarding the AI-powered technique including one of its standout features to adapt to field conditions.
“These findings indicate the significant potential and viability of this AI-driven mid-infrared spectroscopy technique as an affordable and malaria-scalable screening tool in low-resource settings,” they stated.
“In this study, the approach was successfully adapted to field use, effectively predicting natural malaria infections in blood samples from a population-level survey in Tanzania. With additional field trials and validation, this technique could significantly enhance malaria surveillance and contribute to accelerating malaria elimination efforts.”
Ifakara scientists lead the study
The research was led by Issa Mshani, a scientist at Ifakara Health Institute, with Fredros Okumu and Francesco Baldini, also from Ifakara, along with Simon Babayan from the University of Glasgow co-supervising the work equally.
Other key contributors from Ifakara include Frank Jackson, Rehema Mwanga, Prisca Kweyamba, Emmanuel Mwanga, Mgeni Tambwe, Lorenz Hofer, Doreen Siria, and Sarah Moore. Mario González-Jiménez and Klaas Wynne, from the University of Glasgow, also participated in the study.
Read the publication here.