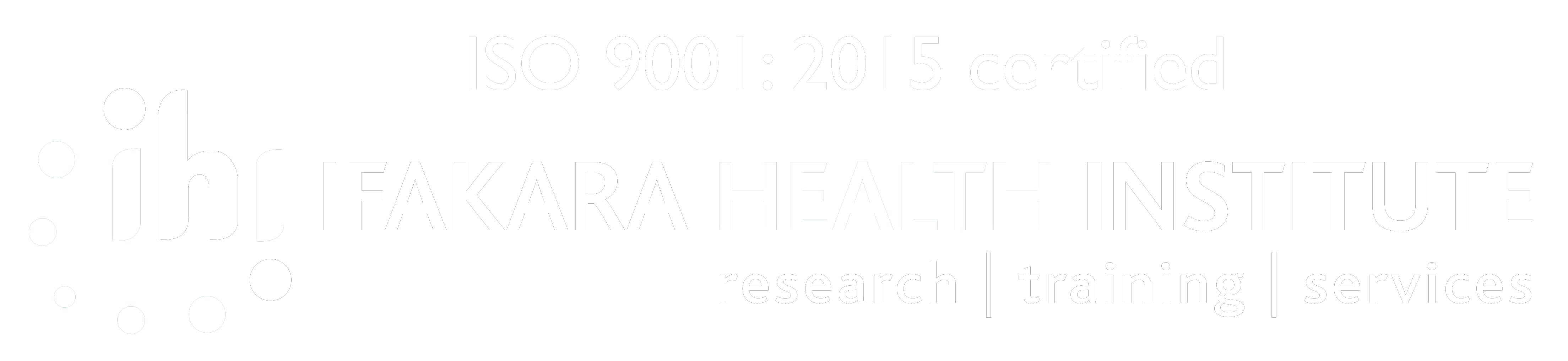
MACHINE LEARNING: Leveraging AI to predict malaria outbreaks
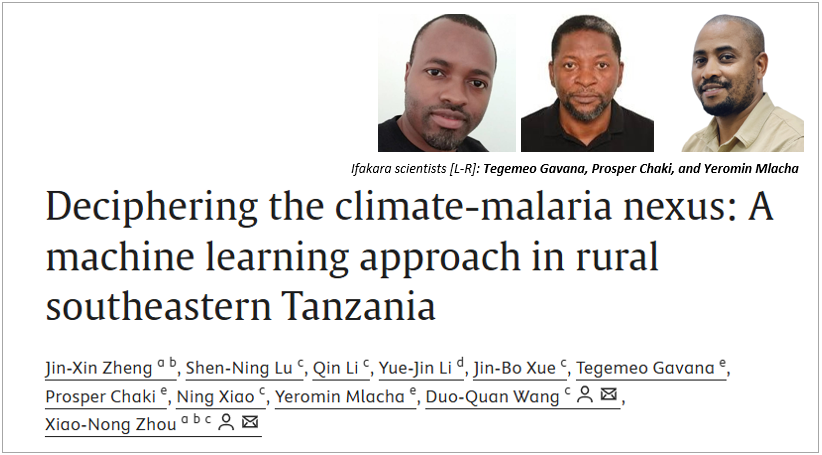
What if malaria outbreaks could be accurately predicted and prevented before they occur? A groundbreaking study has demonstrated the potential of using advanced machine learning techniques and climate data to achieve this.
The research highlights how climate factors such as rainfall and temperature when analyzed using machine learning, can predict malaria incidences and guide more targeted interventions.
The study, led by scientists from China and Tanzania, highlights how environmental and meteorological factors significantly influence malaria transmission. Published in the Public Health Journal, the study provides a framework for data-driven strategies to address one of the region's most persistent public health challenges. Contributors included scientists Tegemeo Gavana, Prosper Chaki and Yeromin Mlacha from the Ifakara Health Institute.
Malaria drivers uncovered with machine learning
Conducted between January 2016 and October 2021, the study focused on the southeastern Tanzanian districts of Kilwa, Rufiji, and Kibiti. By analyzing environmental factors such as rainfall, temperature, and vegetation, the team uncovered critical drivers of malaria transmission.
Using a machine learning model called Extreme Gradient Boosting (XGBoost) and a tool for interpreting its results, SHapley Additive exPlanations (SHAP), the scientists identified the most influential drivers of malaria transmission.
They found that malaria cases peaked during rainy seasons, underscoring the importance of rainfall for mosquito breeding. Lagged climate data—conditions from the previous month—proved essential for accurate predictions of malaria trends, demonstrating how past weather patterns influence mosquito breeding and disease trends. Among the districts studied, Kibiti recorded the highest malaria incidence, with over 15,000 cases during the study period.
“Our study demonstrates the utility of machine learning and explainable SHAP in malaria epidemiology, providing a data-driven framework to guide targeted, climate-informed malaria control strategies. By capturing seasonal and climate-linked risks, these methods hold promise for enhancing public health planning and adaptive response in malaria-endemic regions.” the scientists noted.
How the study advances malaria prevention
The study provides a clear roadmap for improving malaria prevention. It demonstrates the effectiveness of machine learning in revealing the complex interactions between climate factors and malaria transmission in southeastern Tanzania.
By applying machine learning to analyze the complex relationships between climate factors and malaria transmission, public health officials can focus on optimizing intervention timing like insecticide spraying and bed net distributions to align with peak transmission seasons. Additionally, resources can be directed to high-incidence areas like Kibiti, to maximize impact and effectively curb malaria cases.
A step toward malaria elimination
The findings represent a significant step toward reducing malaria cases. By enabling more accurate outbreak predictions and smarter interventions, the data-driven approach empowers health programs to be both efficient and effective.
As the fight against malaria continues in Tanzania, leveraging these tools will be instrumental in achieving its 2025 goal of reaching pre-elimination status, underscored the scientists.
Contributions from Ifakara scientists
This study was conducted by a collaborative team of scientists from China and Tanzania. Among the contributors were Tegemeo Gavana, Prosper Chaki, and Yeromin Mlacha from the Ifakara Health Institute. The Chinese team included researchers from the Chinese Center for Tropical Diseases Research and Shandong First Medical University & Shandong Academy of Medical Sciences.
Read the publication here.