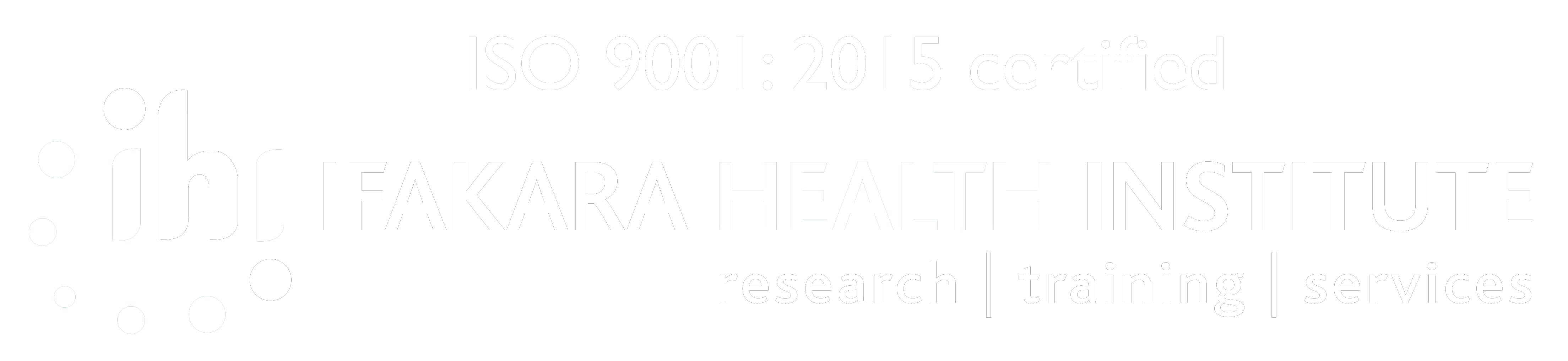
MALARIA WATCH: Drones and AI to improve mosquito surveillance
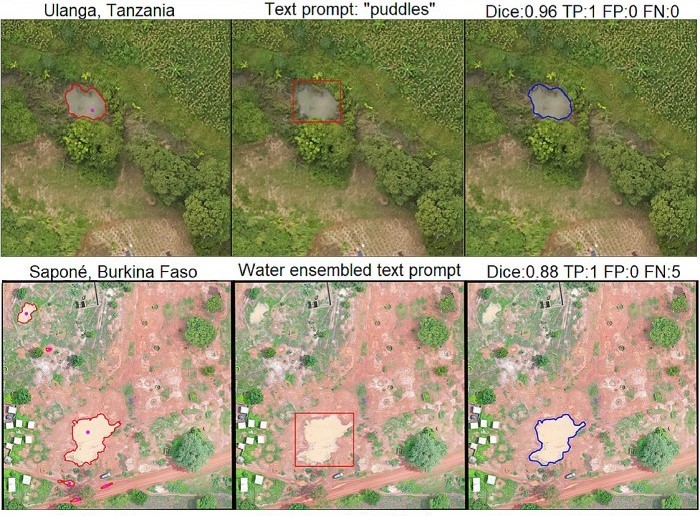
A group of scientists from Europe, Africa, and Asia, among them from Ifakara Health Institute, have underlined the potential benefits of using advanced technical tools like drones and machine learning models to improve surveillance of mosquito-borne diseases.
The adaptation of these innovative tools can help to effectively monitor and manage mosquito populations; however, further evaluation is required to refine their accuracy and applicability, particularly for use in rural areas.
Enhancing vector surveillance in a changing climate
In their paper published on the International Journal of Health Geographics, the scientists highlight the urgency of leveraging these advanced tools to improve vector surveillance methods in response to the challenges of climate change, which continue to alter weather patterns and expand the geographical reach of mosquito-borne diseases.
Pairing technologies for efficacy
According to scientists, drone technology, when paired with other smart models like the Segment Anything Model (SAM), an image segmentation model, can quickly identify important features like water bodies and buildings from drone images.
This capability can find important details in an area quickly such as locating mosquito breeding sites and therefore plan disease control measures more efficiently by analyzing drone images from various geographical locations.
“Recent models such as SAM can potentially assist manual digitalization of imagery by vector control programs, quickly identifying key features when surveying an area of interest,” they noted adding, “However, accurate segmentation still requires user-provided manual prompts and corrections to obtain precise segmentation.”
Using the meta AI Segment Anything Model (SAM)
SAM, developed by Meta AI, is a generalizable trained model capable of extracting image features across various domains. This technology offers novel opportunities for analyzing high-resolution drone imagery collected worldwide. By utilizing advanced machine learning algorithms, SAM automates the segmentation process, reducing the manual effort typically required.
For this study, the scientists tested SAM in malaria-endemic regions of Africa, South America, and Southeast Asia, focusing on identifying critical land cover classes such as water bodies and human settlements.
The findings demonstrated the potential of SAM in aiding the manual digitalization of imagery. However, the performance varied significantly depending on the specific objects, landscape characteristics, and selected words.
“Foundation models, such as SAM, have shown potential to rapidly segment and identify key land classes from high-resolution imagery, enabling the targeting of disease control activities... While these models can be a valuable tool to support the analysis of high-resolution imagery, there is still a need for manual corrections and user input,” noted the scientists of the findings.
Challenges in the efficacy of models
Despite the promising results of SAM, the study also underscores challenges that remain in ensuring the accuracy of segmentation models, particularly regarding specific landscape characteristics and features of the imagery. For instance, big objects like large water bodies and urban houses are segmented more effectively than smaller or irregularly shaped objects such as puddles or rural rounded, highlighting the need for further refinement.
To further improve the efficacy of segmentation models, the scientists recommend doing more research and evaluation. This will help to improve the models’ performance and ensure their robustness for reliable use, especially for applications in rural areas.
Ifakara scientists in the study
Two Ifakara Health Institute scientists contributed to the study. They include Najat Kahamba and Fredros Okumu.
They worked alongside colleagues from the University of Glasgow, Sorbonne Université, Institute du Cerveau, Centre National de Recherche et de Formation sur le Paludisme, Universidad Peruana Cayetano Heredia and the National University of Singapore and National University Health System.
Read the publication here.