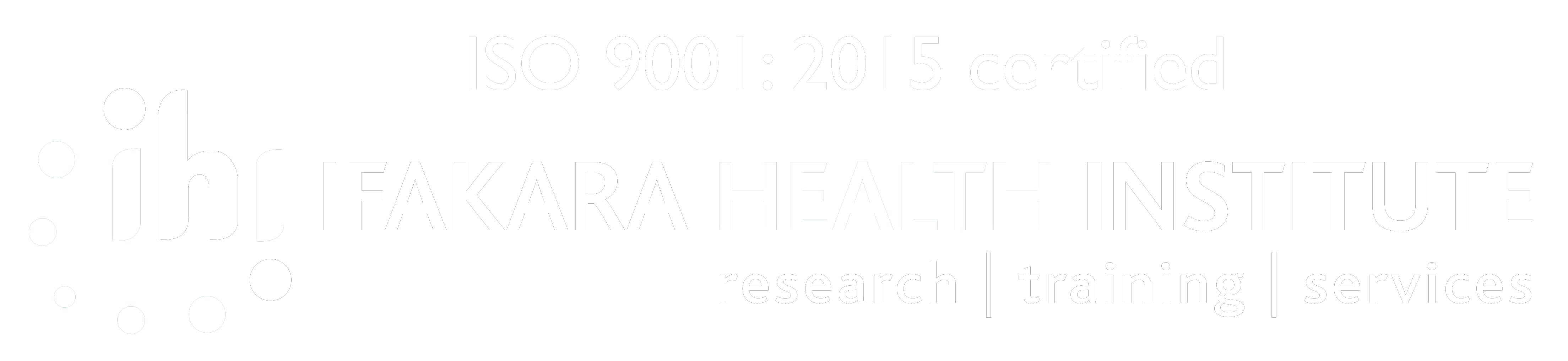
DIGITAL HEALTH: Leveraging AI in epilepsy diagnosis
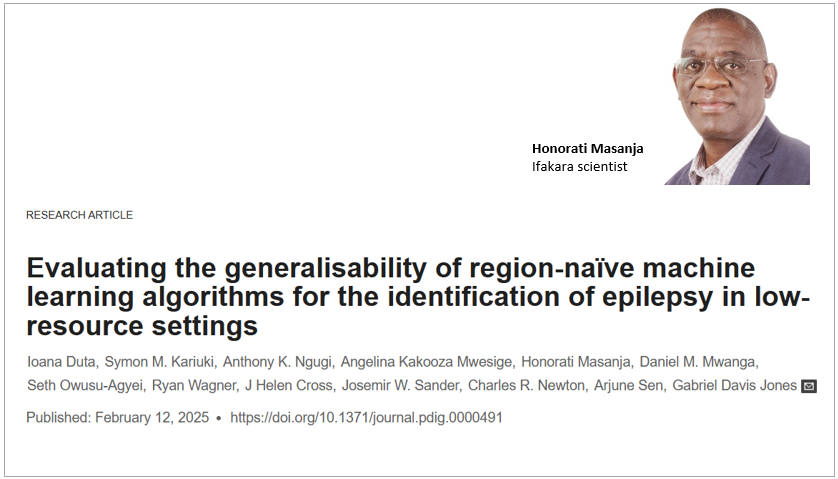
Machine learning has great potential to improve epilepsy diagnosis in low- and middle-income countries (LMICs), where access to specialists is often limited due to constrained healthcare resources and stigma surrounding the condition. However, a recent study published in PLOS Digital Health underscores the need to consider local contexts when developing AI-driven solutions.
The study was led by a group of scientists from the UK and Africa, including Ifakara Health Institute scientist Honorati Masanja. The research team explores the use of machine learning (ML) models to assist in early screening and diagnosis. However, the findings highlight a crucial issue: these models may not perform as effectively when applied in different regions.
Testing machine learning models across regions
The scientists examined how well ML models trained in one region perform when applied elsewhere. They used data from the Study of Epidemiology of Epilepsy in Demographic Sites, which includes clinical and demographic information from five sub-Saharan African locations. The team built predictive models for epilepsy diagnosis, first training them on data from individual sites and then testing them on other sites.
Key findings
The study found that while ML models performed well within the regions where they were trained, their accuracy declined when tested in different locations. Each region had distinct model parameters and diagnostic patterns, making it difficult to apply a one-size-fits-all approach.
When models were trained using data from multiple sites, their ability to work across regions improved, but their performance within specific regions slightly decreased.
“We demonstrate that, when developing models for epilepsy diagnosis, data collected from one site cannot naïvely be used as representative for all other sites where a model could be deployed. Given the needs of LMICs, ML models can be leveraged to have a significant impact,” noted the scientists.
“We recommend applying models in target regions and updating them as necessary. We also caution against generic deployment of models developed and tested in one region into a new area without careful, thorough testing and authentication,” they added.
Why do these differences matter?
The variations in model performance highlight how cultural, social, and medical factors influence epilepsy diagnosis. For example, differences in how symptoms are reported, how patients seek care, and local healthcare practices all affect how machine learning models interpret data. This makes it essential to tailor models to specific local contexts rather than assuming a single model can work universally.
Recommendations to adapt AI for real-world impact
The scientists emphasize the importance of adapting ML models to the local environments where they will be used. They recommend training models with diverse data by incorporating information from multiple regions to improve generalizability.
Additionally, they suggest adjusting models to reflect cultural and healthcare-specific nuances to enhance accuracy. Thorough evaluation before deployment is also crucial, ensuring that models are rigorously tested in new regions before being implemented in clinical settings.
“Machine learning-driven clinical tools are becoming more prevalent in low-resource settings; however, their general performance across regions is not fully established. Given their potential impact, it is crucial models are robust, safe and appropriately deployed.”
Furthermore, the scientists recommended future studies to investigate deep transfer learning models, which have demonstrated the ability to generalize across different datasets stating, “Incorporating such techniques could further enhance the robustness and applicability of epilepsy diagnostic tools in LMICs.”
Read the publication here.